AI can help stressed retailers - Let us guide you how
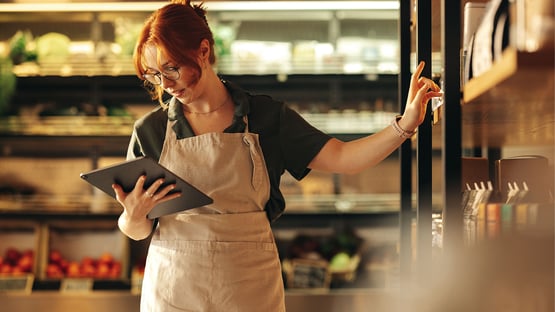
For many retailers, AI remains a vague concept that could potentially help us in the future. But AI is already here and in uncertain economic times, it can help retailers make more accurate decisions. Pricer’s AI expert, Serge de Gosson de Varennes, lists the pitfalls of the field and offers advice to retailers who are ready to take the next step.
It has always been a struggle in retail to predict future sales, because the methods are all based on historical sales figures, and not enough on other, equally relevant factors. These methods are based on trying to detect patterns and trends in earlier data and then hoping they will repeat in the future.
Missing external factors
Unfortunately, these models lack accuracy because they fail to consider important external factors, like demographic changes, inflation or competitors’ prices. It simply hasn’t been possible to create more advanced models that could also take these factors into account and provide better accuracy.
In the past year, it has become clearer than ever just how vulnerable retailers are to these external factors, with many struggling with everything from changing logistics chains to sharp fluctuations in the cost of raw materials.
Abstaining is more expensive
Retailers must therefore increasingly use the advances in artificial intelligence and machine learning.
Unlike traditional methods, it is now possible to make better forecasts at a reasonable price.
When it comes to what factors can be baked into the models, new AI models have few limitations. They give retailers a much more accurate picture. But it is important to pay attention in order to avoid possible pitfalls along the way.
Five pitfalls
- AI models require large amounts of data to be reliable. If the model training incorporates too little data, the forecasts will be worse. But these bigger data sets are usually available these days.
- Be sure your data isn’t biased, for example, that it isn’t heavily weighted toward a certain category, or over-representing a certain kind of customer. This could distort your model.
- Avoid over-adaptation. This is when too much data has been presented in the model training, causing it to over-adapt to this particular data and making it harder to make forecasts from your new data.
- Have access to knowledgeable specialists. Creating reliable models is challenging without a deeper understanding of the underlying math and statistics.
- Have the right expectations. It is often tough to know why you end up with the forecasts you get, even when they are correct. This is why you need people with industry knowledge to determine whether the outcomes are reasonable and to contribute to the understanding of underlying factors.
Not just for a select few
In a sense, AI has been democratized: no longer is it only global retail giants that can afford to invest in AI.
Many of the tools are built on open-source technologies and much of the existing expertise for storing data etc. can also be used to work with AI. However, it’s important to remember that an investment in AI should be seen as ongoing, rather than just an initial cost, because the models need to be maintained, trained and retrained on your data.
Despite these pitfalls, AI offers massive potential for retail. For far too long, forecasting models have become obsolete before they were even used. 2023 is set to be messier than many previous years, with continued price increases and conflicts in the area, as well as disruptions to logistics chains. AI is therefore needed more than ever and it is time for retailers to take action.
Published on May 24, 2023